This content is provided in partnership with Tokyo-based startup podcast Disrupting Japan. Please enjoy the podcast and the full transcript of this interview on Disrupting Japan's website!
Japan is lagging behind in AI, but that might not be the case for long.
Today we sit down with Jad Tarifi, current founder of Integral AI and previously, founder of Google’s first Generative AI team, and we talk about some of Japan’s potential advantages in AI, the most likely path to AGI, and how small AI startups can compete against the over-funded AI giants.
It’s a great conversation, and I think you’ll enjoy it.
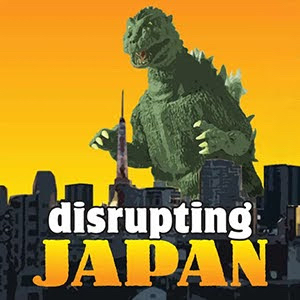
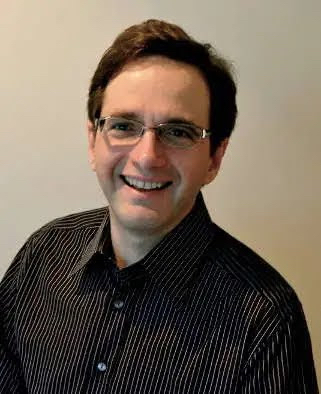
***
Transcript
Welcome to Disrupting Japan, Straight Talk from Japan’s most innovative founders and VCs.
I’m Tim Romero and thanks for joining me.
Japan is lagging behind in AI, but that was not always the case. And it won’t necessarily be the case in the future.
Today we sit down with Jad Tarifi, current founder of Integral AI, and previously founder of Google’s first generative AI team. We talk about his decision to leave Google after over a decade of groundbreaking research to focus on what he sees as a better, faster path to AGI or artificial general intelligence. And then to super intelligence.
It’s a fascinating discussion that begins very practically and gets more and more philosophical as we go on.
We talk about the key role robotics has to play in reaching AGI, how to leverage the overlooked AI development talent here in Japan, how small startups can compete against today’s AI giants, and then how we can live with AI, how to keep our interest aligned.
And at the end, one important thing Elon Musk shows us about our relationship to AI. And I guarantee it’s not what you, and certainly not what Elon thinks it is.
But you know, Jad tells that story much better than I can. So, let’s get right to the interview.
Interview
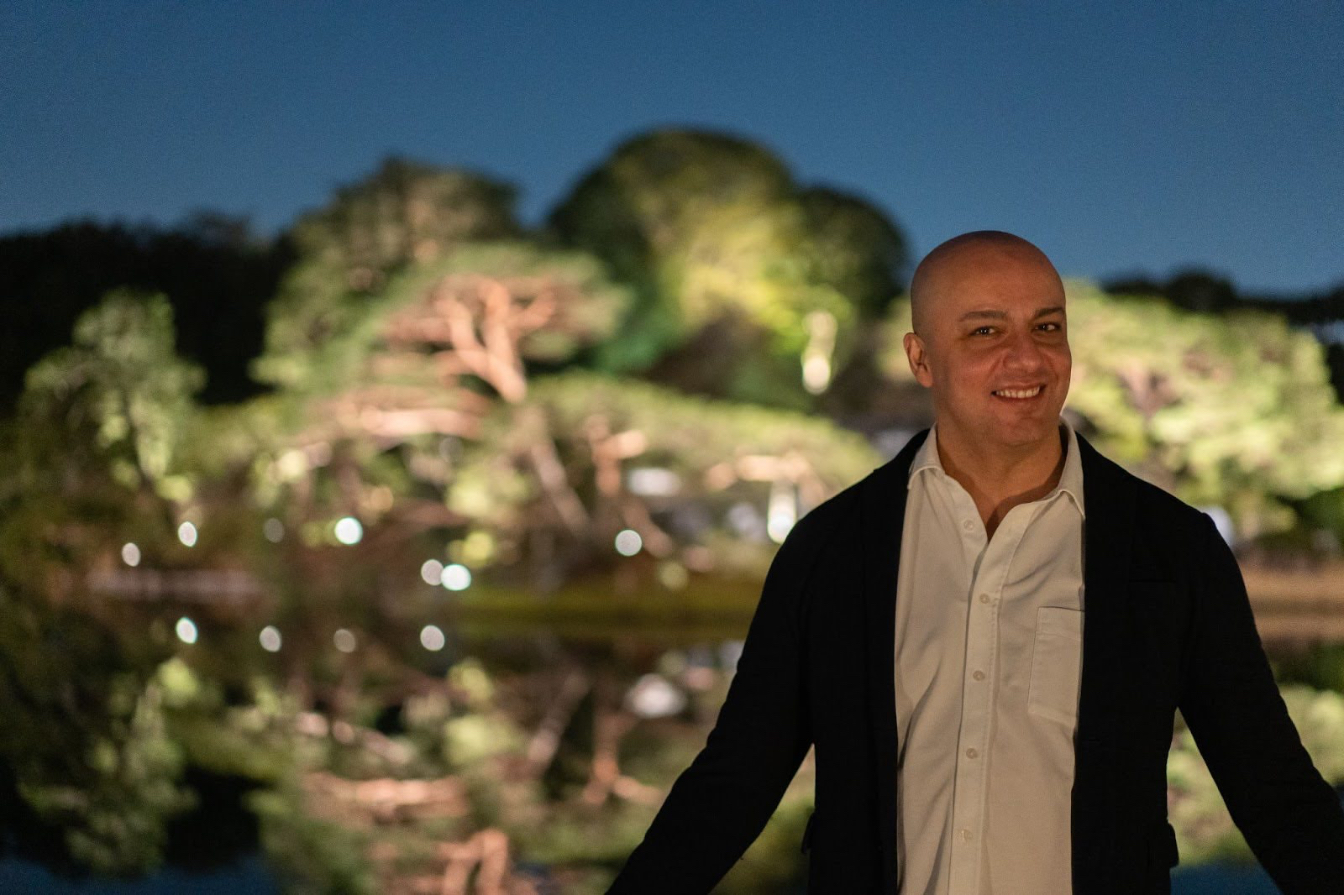
Tim: I am sitting here with Jad Tarifi, founder of Integral AI, so thanks for sitting down with me.
Jad: Thank you.
Tim: Integral AI, you guys are “unlocking, scalable, robust general intelligence.” Now that’s a pretty big claim, so let’s break that down. What exactly are you guys doing?
Jad: So, when we look at generative AI models right now, they usually operate as a black box. And because they have minimal assumptions on the data, they have to do a lot of work and they tend to be inefficient in terms of the amount of data they need and the amount of compute. We’re taking a different approach that’s inspired by the architecture of the neocortex, which roughly speaking follows a hierarchical design where different layers produce abstractions and then feed into higher layers that create abstractions of abstractions and so on.
Tim: Okay, so this is not an LLM architecture or is this a kind of LLM architecture?
Jad: When people talk about LLM, usually they talk about auto regressive transformer networks. So this would be a different type of architecture than that. However we can use transformers or other models like diffusion models as building blocks within that overall architecture.
Tim: It’s interesting that you took a different path than LLMs because you’re not new to AI. You led teams at Google for what? Nine years or so where you were working with transformer architecture. So, you know this technology deeply. What made you decide to not only leave Google and start a new startup, but to leave the LLM path and pursue a different technological architecture?
Jad: So, this all goes back to my PhD where we were exploring how the neocortex could work from an algorithmic perspective. And in fact, when I started the first generative AI team at Google, we were targeting how to have models that can imagine new things, which what we call generative AI right now. Transformers was one of the very exciting, scalable architectures to do so, but there were clearly limitations there that I cared about deeply because I do care about these models affecting the real world, and there was a bottleneck there in terms of reliability and in terms of efficiency. And from my work on the architecture of the neocortex, it was clear that there is a path that goes beyond the current models and I could pursue that path at Google, but it also felt that there’s a new class of applications that are going to be unlocked to have nothing to do with search that more about the physical world robotics movement, real time user interfaces, all of those exciting things that felt a little bit outside the box. And so it felt like it’ll be good to have a new company with a blank slate so we can move fast and make an impact.
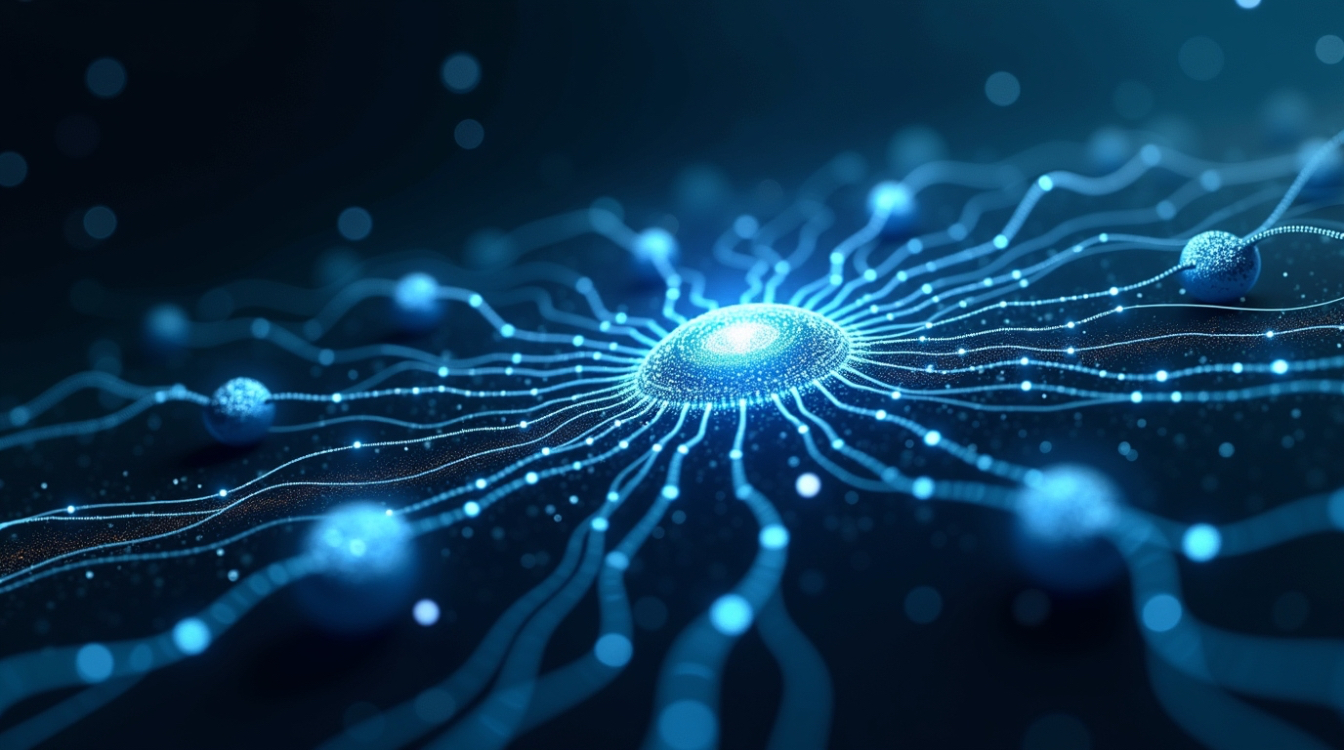
Tim: Integral AI, let’s see, you guys launched in 2021, right? So this was a solid year before, well before generative AI became mainstream. So, has that prediction played out? So, generative AI in the last two years has increased significantly in accuracy and reliability, but do you think it’s going to hit a wall or has hit a wall in terms of how accurate it can be?
Jad: No, I don’t think generative AI will hit a wall at all. As someone who is one of the founders of generative AI, I think the sky’s dilemma, I think we are going to go to general intelligence and beyond human level all the way to super intelligence. In fact, I think the transformer architecture will continue to improve, but the rate of return on general pre-training of these architectures have reached diminishing returns right now. So, you need to spend 10 times more energy, 10 times more data to get the next step up. And if you give me infinite energy, infinite data, then I can do anything. But we are already seeing our models can do much better with far less resources and they have better scalable qualities. So you think about it as the slope of your scaling exponential.
Tim: So, I appreciate the thought that LLMs aren’t going to hit a wall, but if we do have that kind of a scaling issue, we can theoretically come up with 10 times more compute. But is there 10 times more data and is it, is it like quality data? I mean, sure we can train LLMs on YouTube videos and TikTok, but I’m not sure we really want to use those.
Jad: I’ll answer that in three different ways. One is by expanding to modalities. As you mentioned, YouTube vision is largely under explored. Just training on all the text on the internet is not enough. There’s a lot of other data sources including proprietary data sources, but also multimodal data like vision, like sound and all that stuff. But even that has fundamental limits. The next thing is about actually having humans create new data. And I think there’s a lot of ethical issues there. You know, a lot of the data is creating in poor countries, people who are underpaid. A lot of the labs are exploring this strategy and I think to some degree there’s some success there, but I don’t see it as entirely scalable long term. Third and most promising approach is the new paradigm of test time scale. So, these models can look at data reason and then generate new reasoning chains or plans, thereby creating better and better data for themselves. So, there’s this cycle in psychology, it’s system one and system two thinking. So when we think, when we make a plan, this plan becomes a new, fresh, high quality data that we can retrain our intuition. And an example would be chess. When you start off a chess, you need to maybe think about every single move. And as you become more experienced, those naturally come to you and you still have to plan, but you’re planning at the higher level. And so there’s this loop system one gives you a better system two, system two gives you a better system one. And so there’s a real sense in which this model can self-boost, trap and create their own data.
Tim: Okay, that makes sense. Let’s get back specifically to Integral AI and the work you guys are doing. So you mentioned the, the importance of multimodality and you and the team are doing a lot of work with robotics and with DENSO Wave.
Jad: Yeah, so ultimately the way AI is going to impact the real world is through taking physical action. And the form that computers take physical action in the world is robotics. So the way we define robotics is any controllable physical tool. So that includes cars, drones, but even elevators. So anything that you can move intelligently would be in our category, a robot.
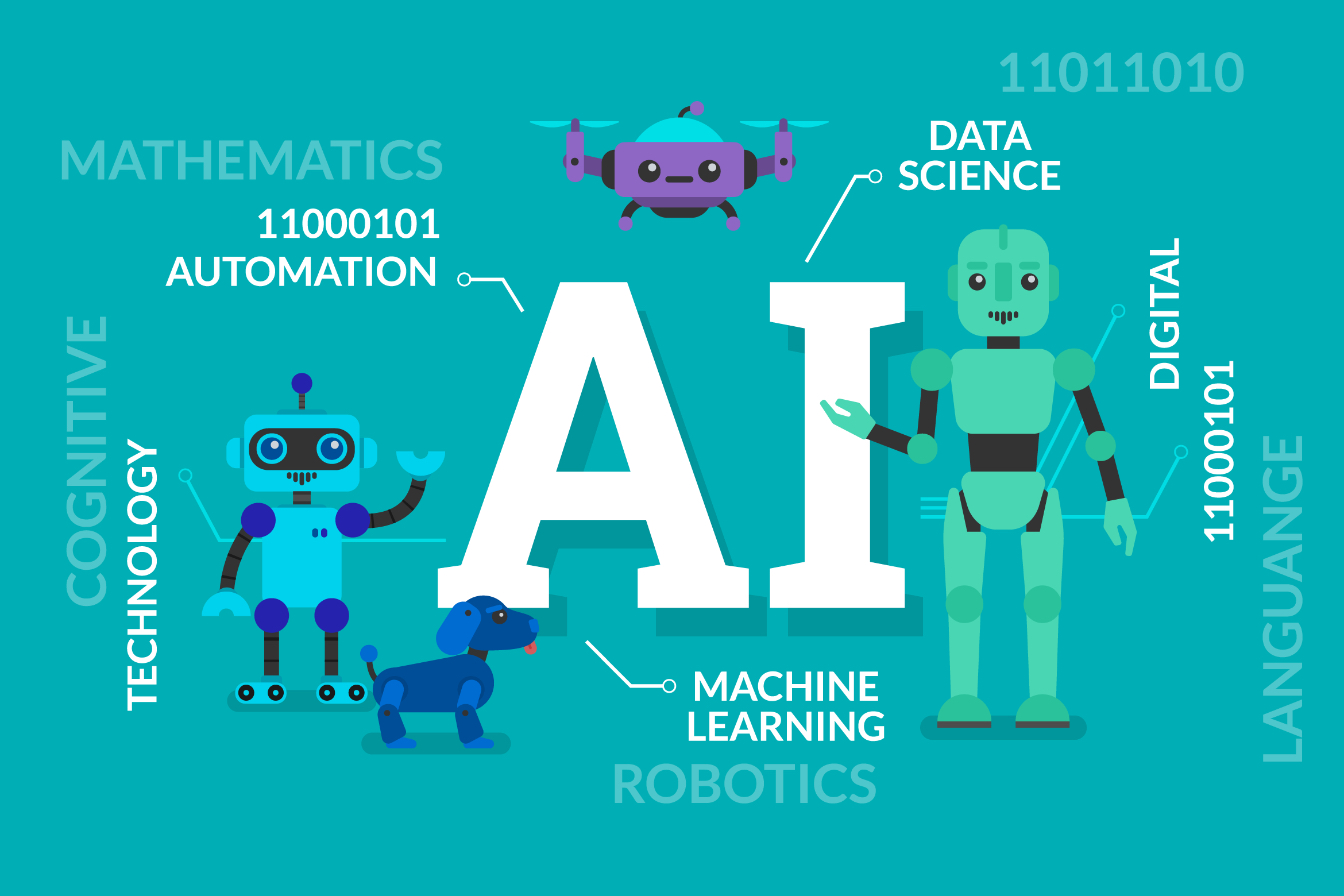
Tim: Well, it sounds like just about anything with a physical manifestation of it would be a robot, anything that can interact with the world.
Jad: Right, that’s our expansive definition of the world. And if you are going to interact with the world, it’s really helpful to understand the world. And the most rich sense for understanding the world is the visual sense. As humans, you know, about 40% of our neo cortex is specialized for vision. So we tend to spend a lot of our energy processing the real world visually, compliments language very well because language is the natural modality for abstract thinking. So there’s the abstract thinking through language and there’s the real world grounding through vision. And so the world of abstraction is already compressed enough that you can get away with a very inefficient algorithm. But as soon as you go to the real world with vision, the data science becomes very large. The complexity becomes much larger in terms of the dimensionality of the problem. And so you need better and better algorithms. Our technology, of course, we can attack these language problems like LLMs, but where it shines is on these harder problems that occur in vision and definitely in the real world. So we thought that robotics is a really nice toy problem or like a specialization to focus the algorithms on. And so we collaborated with DENSO Wave, and many other companies like Honda and we try to find opportunities that this technology can affect their product line now.
Tim: The application of AI and robotics, the fact that there is a physical component to it seems not only difficult, but a unique opportunity for training data in the sense that the robots are interacting with the world, they’re getting their own feedback. They’re in a sense at least theoretically, can explore the world in analogous way to what we do. So in a sense, could they provide their own training data through their own experiences, their own interactions with the world?
Jad: Absolutely correct. I think you identified a key important insight that’s driving us. Our models now are reaching the point where they can collect their own training data. You can ask a question like invent a new drug for me and thinking, go out and do experiments, combine different molecules together, update a theory about how the system or how the drug interaction works, and then do other experiments and have that loop almost like automating the scientific process,. We call that internally active learning. So it’s a process of learning through taking action. It’s something we’re very excited about and we have simple versions of that right now already. We’re actually building up to a much more general version of this that’s going to come out in the next few weeks. The active learning group, we think is the key to achieving AGI.
(To be continued in Part 2)
In the next episode, we’ll cover why Integral AI chose Tokyo as its base, the unique challenges robotics startups face, and what kind of business models can help AI startups compete with the tech giants.
[ This content is provided in partnership with Tokyo-based startup podcast Disrupting Japan. Please enjoy the podcast and the full transcript of this interview on Disrupting Japan's website! ]
Top photo: Courtesy of Disrupting Japan
***
Click here for the Japanese version of the article